Enhancing Customer Insights with Artificial Intelligence:
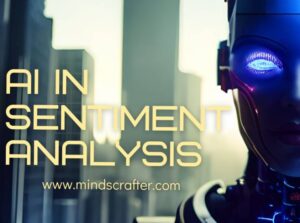
Introduction:
Sentiment analysis is a powerful tool for businesses to understand how customers perceive their products or services. With the rise of artificial intelligence (AI), sentiment analysis has become even more effective in delivering accurate and comprehensive results. In this blog post, we will explore how AI improves sentiment analysis, enabling businesses to gain valuable insights and make informed decisions. Whether you want to enhance customer satisfaction or refine your marketing strategy, these techniques will help you achieve your goals.
-
Harness the Power of Natural Language Processing (NLP):
Utilizing Natural Language Processing (NLP), an AI technique for analyzing and understanding human language can significantly enhance the accuracy of sentiment analysis results. By employing NLP in your analysis, you can identify the tone, context, and intent behind customer feedback, gaining a deeper understanding of their sentiments. This, in turn, allows you to identify areas for improvement in your products or services and tailor your marketing messages to resonate better with your target audience.
-
Enhance Accuracy with Machine Learning (ML) Algorithms:
Machine Learning (ML) algorithms can be employed to improve the accuracy of sentiment analysis results. By training these algorithms on large datasets of labelled data, they can learn to recognize linguistic patterns and nuances that may not be immediately apparent to human analysts. ML algorithms have the advantage of capturing subtle variations in sentiment, including sarcasm or irony, which traditional sentiment analysis techniques might overlook. Moreover, they can identify trends and patterns in customer feedback over time, empowering you to make more informed decisions about your business strategy.
-
Analyze Complex Data with Deep Learning:
Deep Learning techniques offer the capability to analyze complex datasets and unveil patterns that may elude traditional sentiment analysis methods. By employing neural networks to process vast amounts of data, you can identify subtle variations in sentiment and gain a deeper understanding of customer feedback. Deep Learning can also uncover correlations between different types of feedback, providing valuable insights for your business strategy.
-
Leverage Sentiment Analysis APIs for Speed and Accuracy:
Implementing Sentiment Analysis APIs is an efficient way to enhance the accuracy and speed of your sentiment analysis results. These APIs leverage machine learning algorithms to analyze text and determine sentiment. By utilizing an API, you can save time and resources while ensuring more accurate results. Noteworthy Sentiment Analysis APIs include Google Cloud Natural Language API, IBM Watson Tone Analyzer, and Amazon Comprehend.
-
Combine AI with Human Analysis for Optimal Results:
While AI greatly enhances sentiment analysis, it is crucial to acknowledge that it cannot replace human analysis. By combining the strengths of AI and human analysts, you can achieve more accurate and insightful results. AI excels at rapidly analyzing large amounts of data, while humans contribute contextual understanding and interpret nuances that machines may overlook. By harnessing the advantages of both approaches, you can gain a comprehensive understanding of customer feedback and make well-informed business decisions.
Exploring AI in Sentiment Analysis Tools and Resources:
To improve the accuracy and efficiency of sentiment analysis, businesses can leverage AI-powered tools and resources. Here are some excellent AI tools for sentiment analysis:
- Google Cloud Natural Language API: Provides comprehensive sentiment analysis using advanced machine learning models.
- IBM Watson Tone Analyzer: Goes beyond sentiment analysis to identify emotions, tones, and language styles.
- Amazon Comprehend: Offers sentiment analysis in multiple languages with pre-trained models.
- MonkeyLearn: Analyzes customer feedback from various sources and allows customization of sentiment analysis models.
- Hugging Face: Provides access to pre-trained sentiment analysis models or the option to fine-tune models on your own data.
- RapidMiner: A user-friendly data science platform with sentiment analysis functionalities.
- Lexalytics: Offers sentiment scoring, entity-level sentiment, and aspect-based sentiment analysis for valuable insights.
- Aylien: Analyzes large volumes of text data, such as social media posts and news articles, for sentiment analysis.
- Microsoft Azure Text Analytics: Integrates sentiment analysis models into business applications for valuable insights.
- Clarabridge: Enables sentiment analysis from various channels to improve customer satisfaction.
By utilizing these AI-powered sentiment analysis tools, businesses can better understand customer sentiments, make informed decisions, and optimize their overall strategies.
Summary / Conclusion:
Lastly, AI has revolutionized sentiment analysis, enabling businesses to delve deeper into customer sentiments and derive meaningful insights. By incorporating techniques such as Natural Language Processing, Machine Learning algorithms, Deep Learning, and Sentiment Analysis APIs, you can unlock the full potential of sentiment analysis. Remember, the key to success lies in combining AI capabilities with human analysis to achieve the most accurate and valuable results. Embracing AI in sentiment analysis empowers businesses to understand customer feedback better, optimize strategies, and drive overall success.
Removing AI’s threat perception will require lots of efforts..